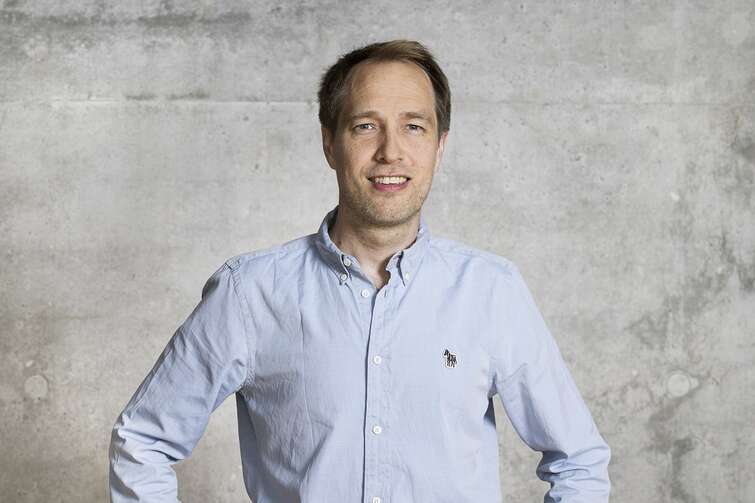
Human Proteome Variation — Rasmussen Group
The group focuses on proteome and genome variation, coding variation and deep learning for integration of genomics, proteomics and clinical data.
From 1 September, 2023 the Rasmussen group is affiliated with the Novo Nordisk Foundation Center for Basic Metabolic Research (CBMR). From 1 January, 2024 the Rasmussen group will be fully employed at CBMR.
The Rasmussen Group focus on computational analysis of large-scale human genome and proteome variation data and couple these with microbiome, health, clinical and registry data. We use these data to generate basic molecular understanding of human health that can guide the development of precision medicine. We therefore work with multi-omics data from the many layers of a cell such as genomics, transcriptomics, proteomics, metabolomics and more to fully characterize the molecular changes in health and disease. Additionally, we have an increasing focus on adding hospital and registry data to define clinically relevant outcomes and their molecular associations.
To investigate such complex biological systems we develop and apply novel bioinformatics and deep learning models for both predictive and investigative analyses. A critical aspect of these approaches is therefore their ability to integrate multiple data types and how they can be used to understand and predict diagnosis or treatment outcomes from all data simultaneously. We try to achieve this by developing state-of-the-art supervised, self-supervised and unsupervised deep learning approaches. Recently, a key focus area of the group has been the development of generative deep learning models for combining molecular and other health measurements and use these to discover new biological hypotheses.
Examples of our recent work include biobank scale deep learning models for analysis of genetic and biomarker data from the UK Biobank cohort (Sigurdsson et al., bioRxiv), integrative analyses of genomics, registry, and hospital data of individuals with psychiatric disorders (Allesøe et al., Science Advances, 2022), and development of generative models for integration and analysis of multi-modal data of people with Type 2 Diabetes (Allesøe et al., Nature Biotechnology, Accepted). Additionally, we collaborate and contribute to developing machine learning models with clinical useability for instance in prediction and diagnosis of liver diseases from plasma proteomics (Niu et al., Nature Medicine, 2022). Finally, our group were the first to develop unsupervised deep learning models for reconstruction of microbial genomes from metagenomics data (Nissen et al., 2021, Nature Biotechnology) which has now been further extended to bacteriophages (Johansen et al., 2022, Nature Communications).
Allesøe et al., Discovery of drug-omics associations in type 2 diabetes with generative deep-learning models. Nature Biotechnology, Accepted.
Sigurdsson et al., Deep integrative models for large-scale human genomics, bioRxiv
Rasmussen group news
Staff list
Name | Title | Phone |
---|